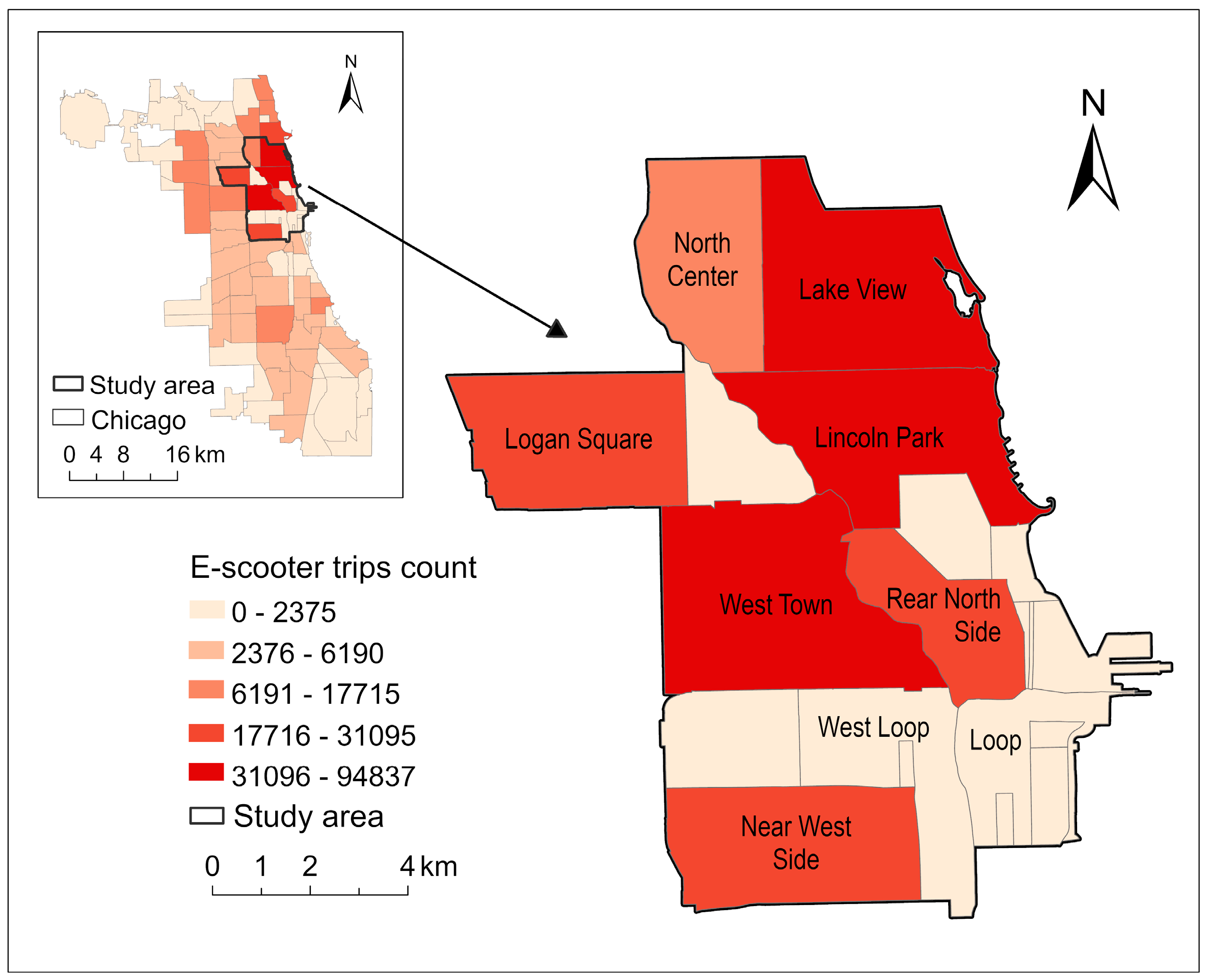
Paper Delivery
Congratulations to Yingqiu Long for her paper "Optimization of Shared Electric Scooter Deployment Stations Based on Distance Tolerance" accepted by ISPRS International Journal of Geo-Information!
Congratulations to Yingqiu Long for her paper "Optimization of Shared Electric Scooter Deployment Stations Based on Distance Tolerance" accepted by ISPRS International Journal of Geo-Information!
Congratulations to Dr. Yang Zhong for his paper "ReCovNet: Reinforcement learning with covering information for solving maximal coverage billboards location problem" accepted by International Journal of Applied Earth Observation and Geoinformation!
Congratulations to Xiao Li for his paper "A Single Data Extraction Algorithm for Oblique Photographic Data Based on the U-Net" accepted by Remote Sensing!
Congratulations to teacher Yueyi Wang for his paper "Dynamic Visual Landscape Assessment of Disused Railway in Complex Terrain: A Study of Jingmen Railway" accepted by IEEE Journal of Selected Topics in Applied Earth Observations and Remote Sensing!
Congratulations to Xiao Li his paper "Development of Geographic Information System Architecture Feature Analysis and Evolution Trend Research" accepted by Journal of Sustainability!
Congratulations to Junyuan Zhou for his paper "A New Approach for Solving Location Routing Problems with Deep Reinforcement Learning of Emergency Medical Facility" accepted by the Geoindustry@ACM SIGSPATIAL 2023 conference!
HISPCRT attended National Key R&D Program Project Discussion on Improving Biodiversity Conservation Assessment Indicators and Effectiveness Monitoring System
Academic Exchange Conference on Maritime transportation digitization and low-carbon transportation
Academic Conference on Research and Application of Big Data in Geographic Flows
Academic Exchange Conference on Maritime transportation digitization and low-carbon transportation
Academic Conference on Research and Application of Big Data in Geographic Flows
White Paper on the Strategic Development of Spatial Data Intelligence in China officially launched at SpatialDI 2024
The Fourth Working Meeting of the Executive Committee of ACM SIGSPATIAL China Chapter (Add new executive members: Shaohua Wang)
The high intelligent spatial computing team visited SuperMap Group to visit and study
HiGISX Group Participated in the Academic Lecture by Prof. Krzysztof Janowicz and Dr. Rui Zhu
HiSpot is an open-source software package developed by the HiGISX team, aimed at solving spatial optimization problems. The software provides multiple solution methods, including mathematical planning solvers, approximate algorithm, and heuristic algorithm, mainly solving location routing problems and facility location problems.
SpoNet is a unified deep learning model used to solve p-median, p-center, and maximal covering location problems. It offers a new approach to solving spatial optimization problems and provides a novel method for addressing large-scale spatial optimization challenges.
GCN-Greedy is a hybrid framework using Graph Convolutional Network and Greedy algorithm for Covering Location Problem. We use GCN network to solve location set covering problem (LSCP) and maximum covering location problem (MCLP). The optimization of the loss function takes into account the characteristics of covering location problems. This model is more accurate and more efficient than classical heuristic algorithms.