- Geospatial optimization
- Deep reinforcement learning
- Remote sensing big data analysis, and integrated applications of digital earth
Haojian Liang
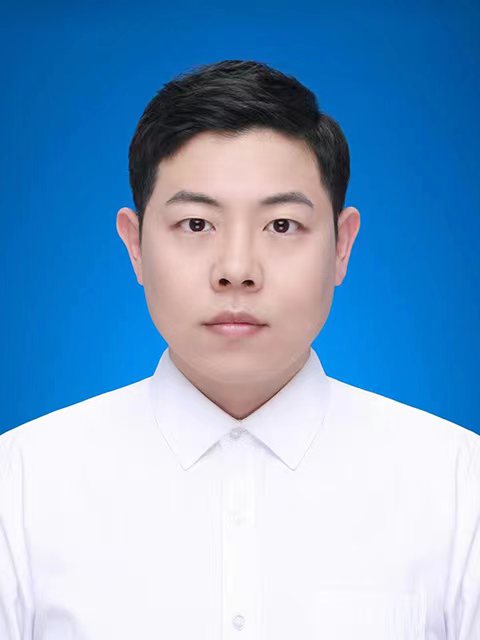
Haojian Liang
Aerospace Information Research Institute, Chinese Academy of Sciences
Special Research Assistant
Biography
Dr. Haojian Liang is a Special Research Assistant at the Aerospace Information Research Institute, Chinese Academy of Sciences. His primary research areas include geospatial optimization, deep reinforcement learning, remote sensing big data analysis, and integrated applications of digital earth. He possesses strong mathematical logic, mathematical modeling skills, programming abilities, and proficiency in English writing. He is well-versed in Python and other development tools, proficient in deep learning frameworks such as Pytorch, and familiar with basic operations in the Linux system.
Research interests
Education
-
2020.07-2024.06, School of Artificial Intelligence, Jilin University, Mathematics, Ph.D.
-
2017.09-2020.06, School of Mathematics, Jilin University, Basic Mathematics, Master's degree
-
2013.09-2017.07, School of Mathematics, Jilin University, Information and Computational Sciences, Bachelor's degree
Experience
-
2024.07-Present, Aerospace Information Research Institute, Chinese Academy of Sciences. Special Research Assistant
Publications
Journal Paper
- Liang H, Wang S*, Li H, et al. Sponet: solve spatial optimization problem using deep reinforcement learning for urban spatial decision analysis[J]. International Journal of Digital Earth, 2024, 17(1): 2299211.
- Liang H, Wang S*, Li H, et al. BiGNN: Bipartite graph neural network with attention mechanism for solving multiple traveling salesman problems in urban logistics[J]. International Journal of Applied Earth Observation and Geoinformation, 2024, 129: 103863.
- Liang H, Wang S*, et al. DeepHullNet: A Deep Learning Approach for Solving the Convex Hull and Concave Hull Problems with Transformer[J], International Journal of Digital Earth.
- Liang H, Wang S*, Li H, Ye H, Zhong Y. A Trade-Off Algorithm for Solving p-Center Problems with a Graph Convolutional Network[J]. ISPRS International Journal of Geo-Information, 2022, 11(5): 270. https://doi.org/10.3390/ijgi11050270
- Zhong Y, Wang S*, Liang H, et al. ReCovNet: Reinforcement learning with covering information for solving maximal coverage billboards location problem[J]. International Journal of Applied Earth Observation and Geoinformation, 2024, 128: 103710.
- Zhang Y, Wang S*, Liang H, et al. Dual hybrid frameworks combining graph convolutional network with decoding for covering location problem[J]. Iscience, 2024, 27(5).
- Chen X, Wang S.*, Li H, Liang H, Li Z, & Lu H. An Attention Model with Multiple Decoders for Solving p-Center Problems. International Journal of Applied Earth Observation and Geoinformation, 2023, 125: 103526.https://doi.org/10.1016/j.jag.2023.103526 https://github.com/HIGISX/AMMD-PC
- Zhao J, Wang S*, Xie B, Wang Z, Liang H, & Lu H. Improved Benders Decomposition Algorithm for City Emergency Service Facility Location. Computers & Operations Research (under review) https://github.com/jinqiuzhao/IBD-of-p-center-and-p-median
- Chen X, Wang S*, Li H, Lyu F, Liang H, Zhang X, & Zhong Y. Ndist2vec: Node with Landmark and New Distance to Vector Method for Predicting Shortest Path Distance along Road Networks. ISPRS International Journal of Geo-Information, 2022, 11(10), 514. https://doi.org/10.3390/ijgi11100514 (SCI)
- Yue J, Long Y, Wang S*, Liang H. Optimization of Shared Electric Scooter Deployment Stations Based on Distance Tolerance[J]. ISPRS International Journal of Geo-Information, 2024, 13(5): 147.
Conference Paper
- Liang H, Wang S*. A New Approach Based on Graph Neural Network for Solving p-center Problems. (Oral, GeoKG&GeoAI 2021)
- Liang H, Wang S*. A Graph Convolution Neural Network Methodology for Solving Traveling Salesman Problem. (Poster,spatialDI 2022)
- Wang S, Liang H*, Zhong Y, Zhang X, & Su C. DeepMCLP: Solving the MCLP with Deep Reinforcement Learning for Urban Facility Location Analytics. 2023 Symposium on Data Science and Statistics. https://doi.org/10.25436/E2KK5V
- Wang S, Liang H*, Zhong Y, Su C, Chen X, Zhou J. DeepSpo: A Deep Learning Framework for Solving Spatial Optimization Problems. (GeoAI 2023, under review)
- Wang S, Zhou J, Liang H*, et al. A New Approach for Solving Location Routing Problems with Deep Reinforcement Learning of Emergency Medical Facility[C] Proceedings of the 8th ACM SIGSPATIAL International Workshop on Security Response using GIS 2023. 2023: 50-53. https://doi.org/10.1145/3615884.3629429
- Wang S, Zhang Z, Su C, Zhou L, Liang H, Wang W. Spatial Optimization Site Selection of Beijing Cainiao Station Based on Multi-Source Geospatial Data. In Proceedings of the 1st ACM SIGSPATIAL International Workshop on Geocomputational Analysis of Socio-Economic Data (GeoSocial '23). Association for Computing Machinery, New York, NY, USA, 20–23. https://doi.org/10.1145/3615892.3628479
- Wang S, Wang R, Su C, Zhou L, Wang W, and Liang H. Optimization of shared bicycle location in Wuhan city based on multi-source geospatial big data[C] Proceedings of the 2nd ACM SIGSPATIAL International Workshop on Spatial Big Data and AI for Industrial Applications. 2023: 43-46. https://doi.org/10.1145/3615888.3627815